MultiMediate 2025
Here, we introduce the different challenge tasks, evaluation methodology and rules for participation.
We provide links to official baseline implementations at the end of each challenge task description. Please be aware that these official baselines are usually just simple approaches. For the state of the art on each challenge task, please consult the challenge leaderboard https://multimediate-challenge.org/Leaderboard/.
Cross-cultural Multi-domain Engagement Estimation
Knowing how engaged participants are is important for a mediator whose goal it is to keep engagement at a high level. The task of engagement estimation involves the continuous, frame-wise prediction of the level of conversational engagement of each participant on a continuous scale from 0 (lowest) to 1 (highest). We provide training- and test datasets from different domains, including variations in language and cultural background as well as different social situations. Participants are encouraged to investigate multimodal as well as reciprocal behaviour of both interlocutors. We will use the Concordance Correlation Coefficient (CCC) to evaluate predictions. The overall performance of a team will be evaluated by taking the average CCC across the four different test datasets.
In the following, we will present the different datasets that are included in the challenge. All of them will be used as test data, some of them are available for training. To take part in the challenge, participants need to submit predictions on all four test datasets.
- NOXI (MultiMediate’23 version): : A dataset of novice-expert interactions recorded with microphones and video cameras, and with frame-wise engagement annotations. This dataset is identical to the engagement estimation challenge in MultiMediate'23 and consists of recordings in English, French and German. Training, validation and test sets are available.
- NOXI (additional languages): This data is used as a test set only and includes four languages that are not part of the original NOXI dataset: two sessions in Arabic, two in Italian, four in Indonesian, and four in Spanish. As a result, this evaluation set tests the ability of participants' approaches to transfer to new languages and cultural backgrounds not seen at training time.
- NOXI+J (additional languages and culture): A novel dataset collected according to the NOXI protocol, but in Japan with Japanese and Chinese speakers. Training, validation and test sets are available.
- MPIIGroupInteraction: We collected engagement annotations on the MPIIGroupInteraction test and validation sets. The validation set with ground truth annotations will be provided to participants to monitor their performance on the out-of-domain task. In addition it may be used as a limited set of training data to develop supervised domain adaptation approaches.
The following Table gives an overview over the datasets used in the cross-cultural multi-domain engagement estimation challenge. Languages covered by each dataset are given in italics, with the respective number of interactions in parentheses.
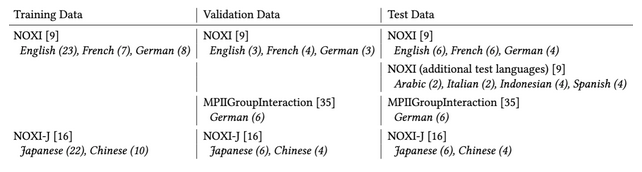
For the cross-cultural multi-domain engagement estimation task, we provide the raw audio and video recordings along with a comprehensive set of pre-computed features for download. These include eGemaps v2, w2vbert2, XLM RoBERTa, OpenFace 2.0 (every frame), OpenPose (every frame), CLIP (768 values per frame, every frame), VideoSwinTransformer (768 values per 16 frames, every 16 frames non-overlapping), VideoMAEv2 (1408 values per 16 frames, every 16 frames non-overlapping), DINOv2+PCA(3) (768x3 values per frame, every 16 frames).
Official baseline approaches are not yet available, but will be published soon. To get started, you can hava a look at the baseline implementations from last year: https://git.opendfki.de/philipp.mueller/multimediate24. We will notify you when the new baselines are available.
Continuing MultiMediate Tasks
In addition to the two tasks described above we also invite submission to the three most popular tasks included in MultiMediate’21-’23.
Bodily Behaviour Recognition
Bodily behaviours like fumbling, gesturing or crossed arms are key signals in social interactions and are related to many higher-level attributes including liking, attractiveness, social verticality, stress and anxiety. While impressive progress was made on human body- and hand pose estimation the recognition of such more complex bodily behaviours is still underexplored. With the bodily behaviour recognition task, we present the first challenge addressing this problem. We formulate bodily behaviour recognition as a 14-class multi-label classification. This task is based on the recently released BBSI dataset (Balazia et al., 2022). Challenge participants will receive 64-frame video snippets as input and need output a score indicating the likelihood of each behaviour class being present. To counter class imbalances, performance will be evaluated using macro averaged average precision. We encourage all participants in the bodily behaviour recognition task to also evaluate their approaches on the related Micro-Action Analysis Challenge (MAC 2025) (https://sites.google.com/view/micro-action). Strong results in both challenges will improve the chances of your papers being accepted. However, please be aware that we do not accept double submissions, i.e. you should submit your paper to only one of the two challenges (either MultiMediate’25 or MAC’25).
Official baseline approaches are available at https://git.opendfki.de/philipp.mueller/multimediate23/-/tree/main/bodily_behaviour.
Backchannel Detection (Multimediate'22 task)
Backchannels serve important meta-conversational purposes like signifying attention or indicating agreement. They can be expressed in a variety of ways - ranging from vocal behaviour (“yes”, “ah-ha”) to subtle nonverbal cues like head nods or hand movements. The backchannel detection sub-challenge focuses on classifying whether a participant of a group interaction expresses a backchannel at a given point in time. Challenge participants will be required to perform this classification based on a 10-second context window of audiovisual recordings of the whole group. Approaches will be evaluated using classification accuracy.
Official baseline approaches are available at https://git.opendfki.de/philipp.mueller/multimediate22_baselines.
Eye Contact Detection (MultiMediate’21 task)
We define eye contact as a discrete indication of whether a participant is looking at another participant’s face, and if so, who this other participant is. Video and audio recordings over a 10 second context window will be provided as input to provide temporal context for the classification decision. Eye contact has to be detected for the last frame of the 10-second context window. In the next speaker prediction sub-challenge, participants need to predict the speaking status of each participant at one second after the end of the context window. Approaches will be evaluated using classification accuracy.
Official baseline approaches are available at https://git.opendfki.de/philipp.mueller/multimediate22_baselines.
Evaluation of Participants’ Approaches
Training, validation, and test data for each sub-challenge can be downloaded at multimediate-challenge.org/Datasets/. We provide pre-computed features to minimise the overhead for participants. For the tasks newly included in this years’ challenge, the test set is now released. Participants can submit their predictions for evaluation at https://hcai.eu/challenges/web/challenges/challenge-page/23/overview.
We will evaluate approaches with the following metrics: accuracy for backchannel detection and eye contact estimation, mean squared error for agreement estimation from backchannels, and next speaker prediction is evaluated with unweighted average recall.
Rules for participation
- The competition is team-based. A single person can only be part of a single team.
- For the cross-cultural multi-domain engagement estimation task, each team will have 5 evaluation runs on the test set.
- For the tasks that were already included in Multimediate’21-23, three evaluations on the test set are allowed per month. In June 2025, we will make an exception and allow for five evaluations on the test set.
- Additional datasets can be used, but they need to be publicly available.
- The Organisers will not participate in the challenge.
- Complete test sets (without labels) will be provided to participants 2 weeks before the challenge deadline. It is not allowed to manually annotate any test data.